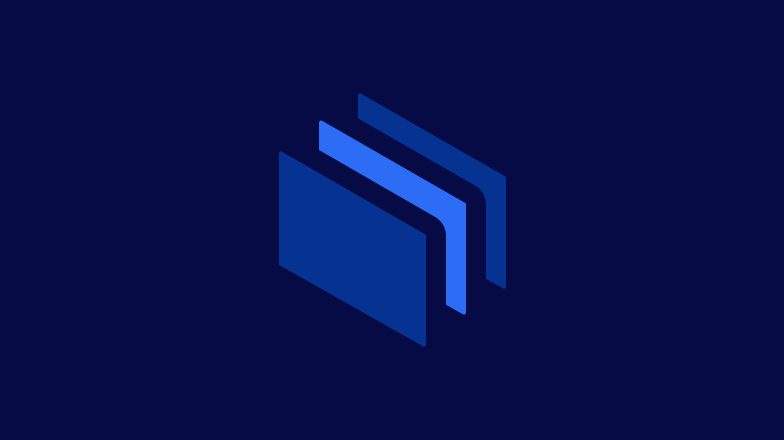
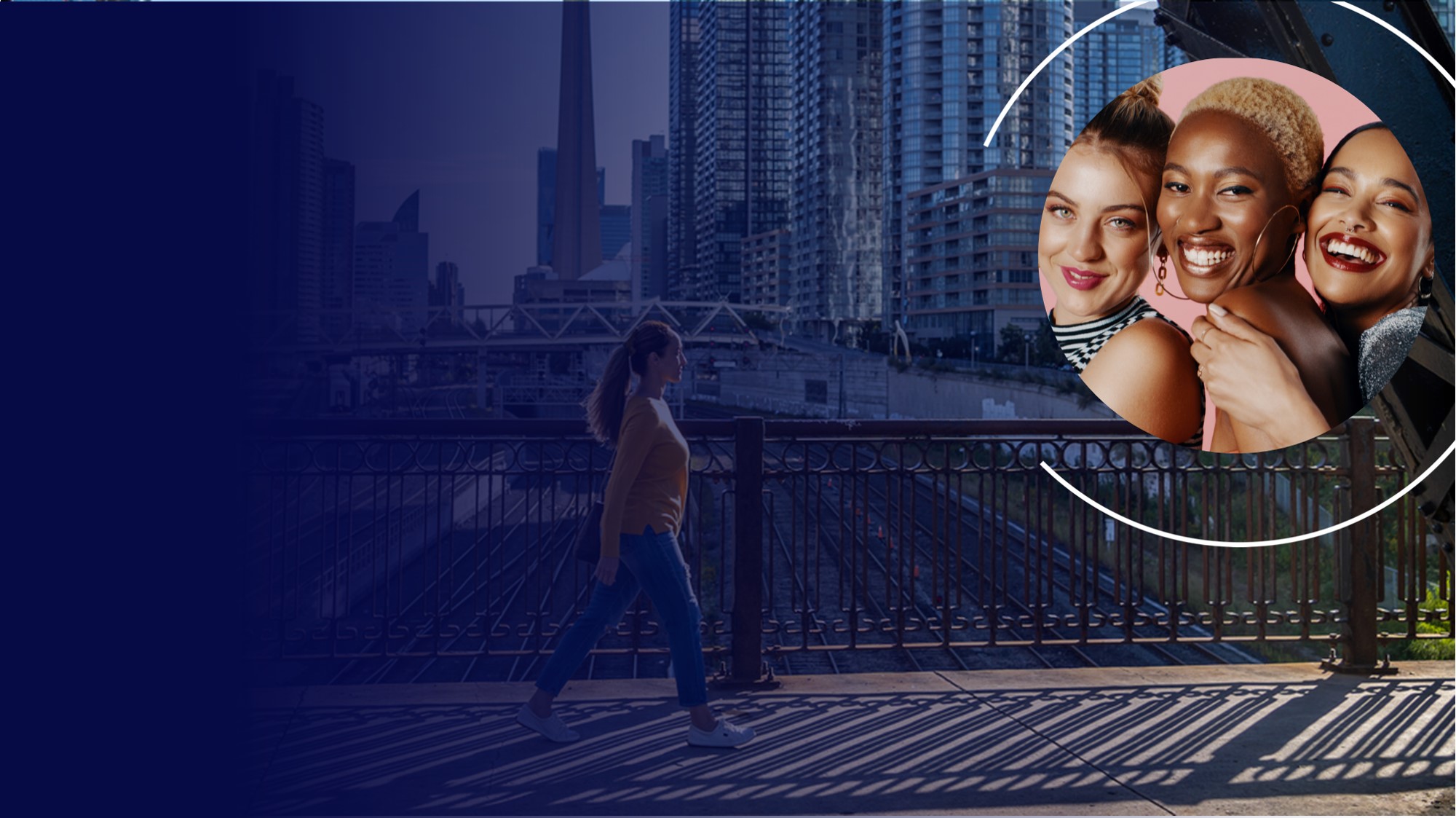
Shaping Success:
A Deep Dive into Women’s Impact on the CPG Landscape
Women’s purchasing behaviors, values, and needs are reshaping the CPG market and challenging businesses to adapt and thrive.
Do you have
the Full View™?
Uncover insights from the world’s largest database of consumer buying behavior. NIQ delivers the most complete and clear understanding of shopper and market trends so that you can know what’s happening, why it’s happening, and what to do about it.
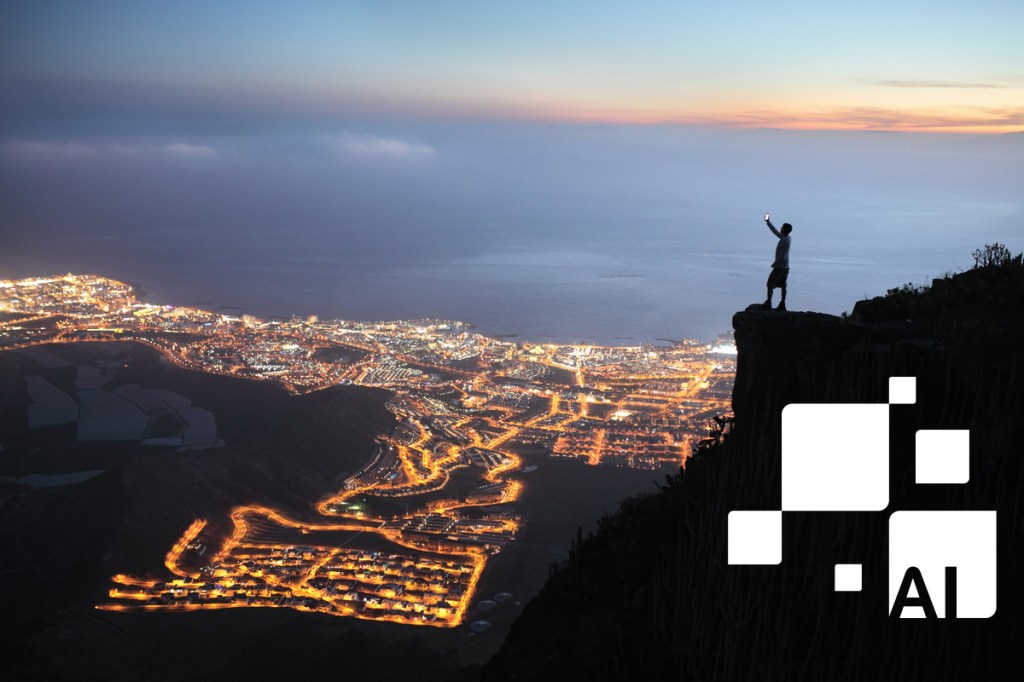
The Full View of
Artificial Intelligence
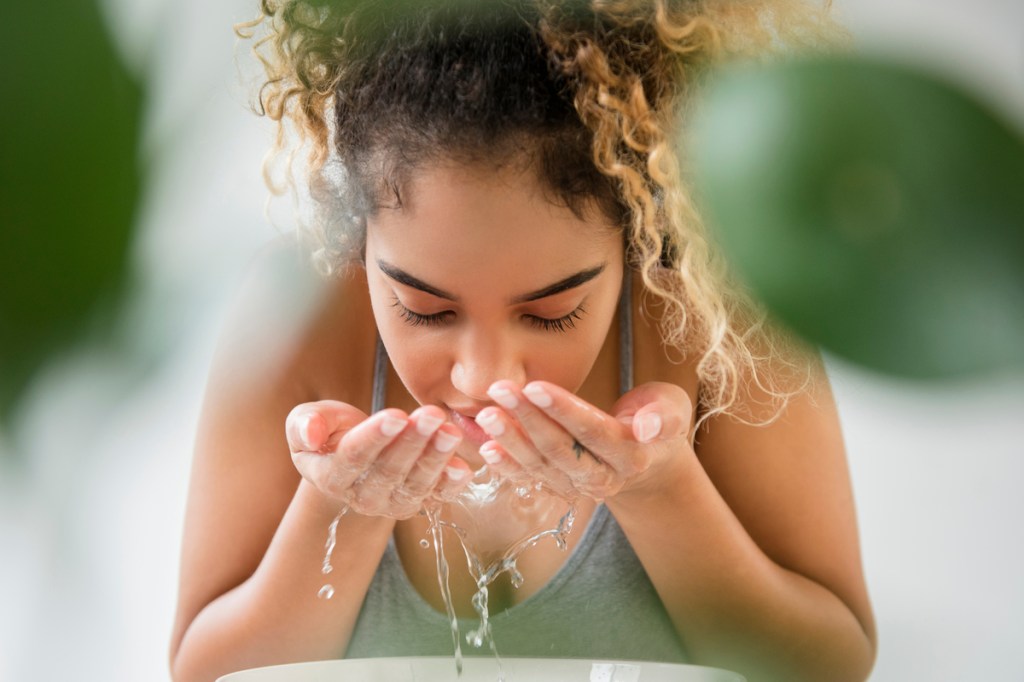
The Full View of
Beauty
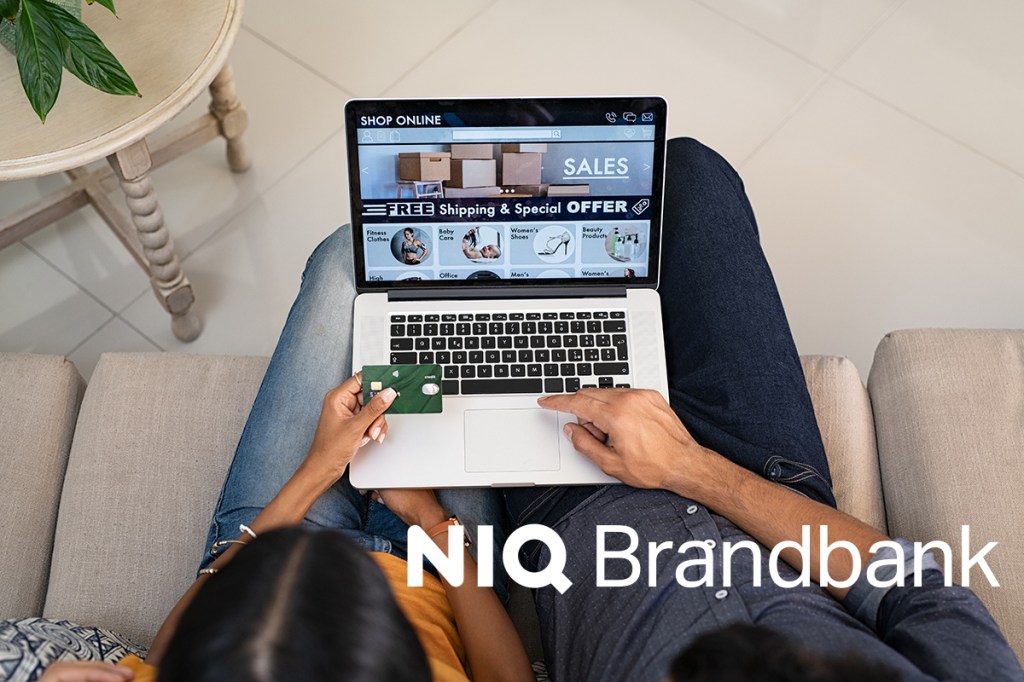
The Full View of
Digital Shopper Experiences
Solution spotlight
Do you have a full view of your sales?
Capture fully integrated online and offline sales data to unlock the value of true omnichannel measurement with NIQ Omnisales.
Solutions
Our solutions uncover the most granular details to inspire the big picture ideas, helping business leaders envision the future and take decisive action.
Diversity & Inclusion
Our century-old legacy holds diversity and inclusion at its core. We win when our people are empowered to be themselves.
Careers
We transform how people understand the world. Your work will redefine what’s possible for both our clients and your career. Join us and change the landscape.
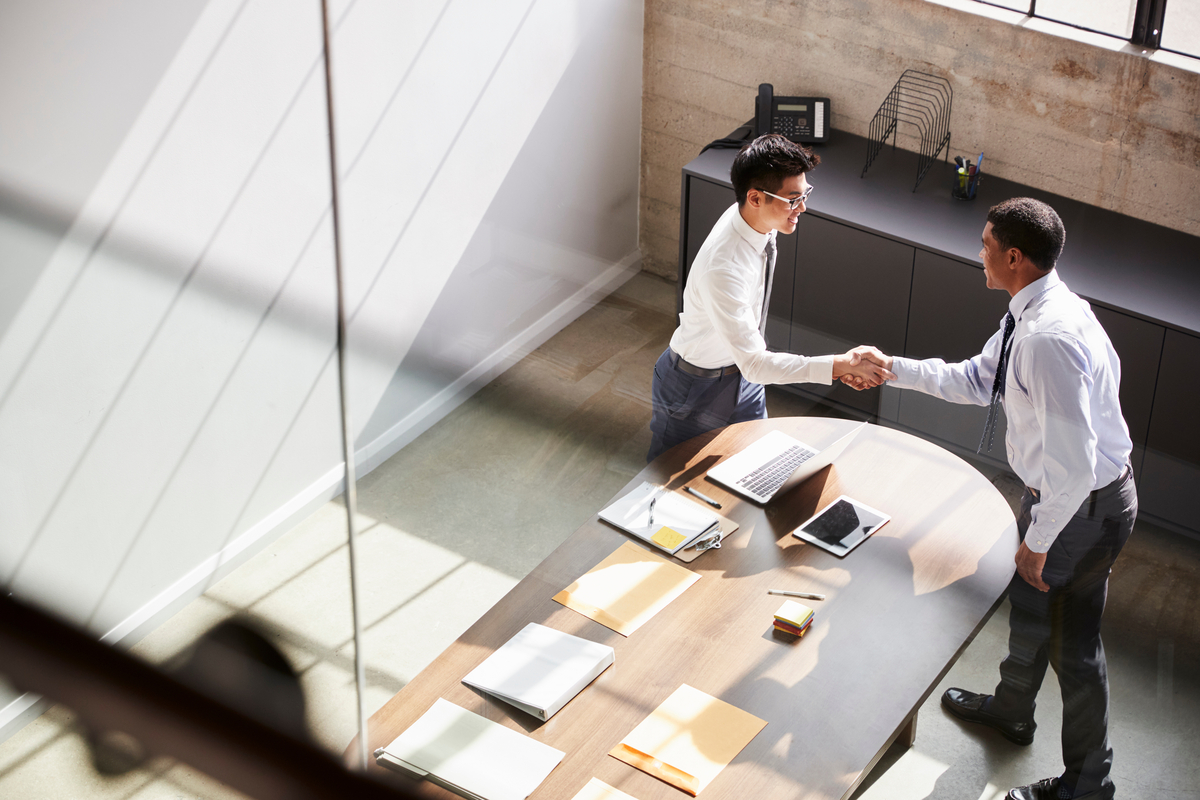
Amplify your business with a reliable partner
The NielsenIQ Partner Network makes it simple to find the right solution for your specific business need. Choose from a network of thoroughly vetted partners and avoid the hassles of data management and third-party agreements.
Stay ahead by staying in the loop.
Don’t miss the latest insights, offerings, and opportunities from NielsenIQ.
By clicking on sign up, you agree to our privacy statement and terms of use.