In recent years, the creation of large single-source datasets has been a major boon to the advertising research industry. At Nielsen Catalina Solutions, we’re combining in-store sales data from millions of households with information on whether or not those households are exposed to any given ad campaign. By examining the sales differential between exposed and unexposed households, we’re able to compute the sales lift generated by thousands of campaigns with great accuracy.*
The ANCOVA (analysis of covariance) model that forms the basis of this test-and-control methodology has been thoroughly tested, and it provides quick and reliable answers to brand managers interested in measuring the effectiveness of a campaign as a whole. But there are occasions when it doesn’t quite fit the bill. Consider, for instance, the case of a campaign that reached such a large audience that it’s nearly impossible to find households that were not exposed to it (see Fig. 1). Where would we find the control group?
To address this challenge, we’ve developed a new methodology called ‘Cognitive Advantics’ (CA). Instead of examining sales lift in aggregate over the course of the entire ad campaign, it analyzes the household sales data at the level of each purchase occasion and takes into account the timing of ad exposures every step of the way—a much more granular look at the data. After all, a household might see an ad, make a purchase, see an ad again for the same brand, and we’d be hard-pressed to say that the second ad had any influence on that particular purchase. Conversely, a household might see an ad, make a purchase two months later, and with so much time in-between, it would be difficult to conclude that the ad exposure was the determining factor behind that purchase.
By analyzing data at the purchase-occasion level, we’re able to take exposure ‘recency’ into consideration—the more recent the ad, the greater the impact. While the effective time window can vary from study to study, we generally look back 28 days from the time of purchase to find one or more exposures to which the purchase occasion can be attributed (see Fig. 2). We’re also able to solve the control group problem because while there may not be many households who haven’t been exposed to the campaign at one point or another, there are generally enough purchase occasions that weren’t influenced by an ad right before the purchase—even among exposed households.
To perform the analysis, the CA methodology takes all relevant variables (purchase history, media consumption, demographics, location, category purchases, etc.), feeds them into a collection of data modeling algorithms and allows the data to pick and combine the models so that the results have the best (i.e., most statistically sound) cross-validation. This is the ‘cognitive’ part in the CA name. The end result is a very powerful tool that relies very little on human intervention and can be deployed at scale.
As the market moves toward real-time, push-button solutions, this is the next evolution in the measurement of advertising effectiveness. The early results are very promising, and we’re looking forward to sharing details, examples and performance benchmarks in a future edition of the journal.
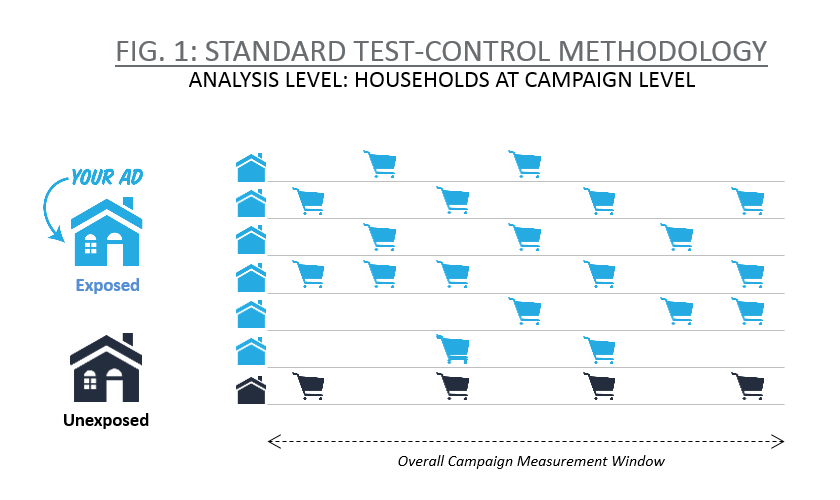
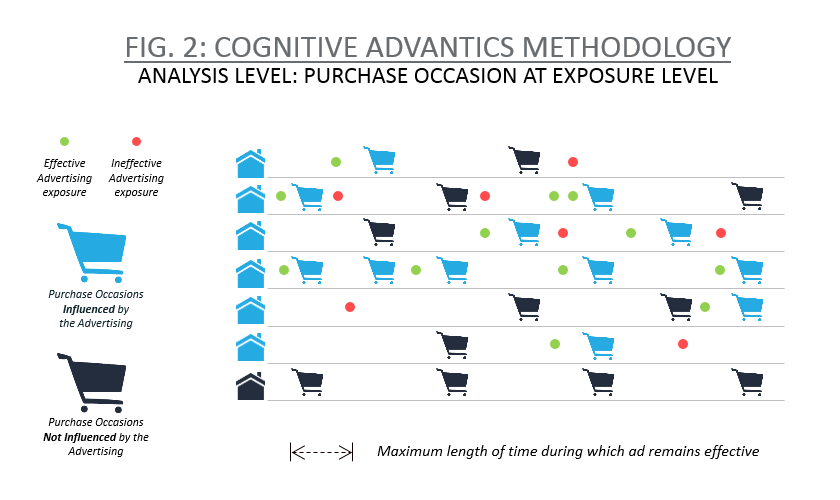
*See details about this method in: Using single-source data to measure advertising effectiveness in VOL 1, ISSUE 2 of the Nielsen Journal of Measurement.